The six most used Data Quality dimensions
Almost every business leader recognises the value of data: for innovation, growth, customer satisfaction, efficiency, and compliance. But data only creates impact when it is trustworthy. That trust depends on Data Integrity: the degree to which data remains accurate, consistent, and reliable across time and systems. Data Integrity ensures your decisions are grounded, not assumptions.
And it all starts with Data Quality. Data Quality lays the foundation for Data Integrity. Organisations that invest in Data Quality create alignment, reduce risk, and unlock data-driven value. But how do you know whether your data is of high quality?
The answer lies in measuring it. Measuring Data Quality involves evaluating key dimensions that reflect how well data supports real business needs. These dimensions help you identify what “good” looks like, based on context, use case, and strategic goals.
In this blog, we focus on six of the most widely used and impactful Data Quality dimensions: accuracy, completeness, consistency, timeliness, validity, and uniqueness. These dimensions are not only essential in practice but also recognised and supported by industry standards such as the DAMA-DMBOK. We explain what each one means, why it matters, and how to measure and improve it.
What is Data Quality?
Data Quality is a quantifiable measure of data’s fitness for use. At its core, it ensures that data meets the needs and expectations of its users. High Data Quality can drive confident business decisions, whereas low Data Quality can lead to financial loss and damage to brand value. Measuring and improving Data Quality involves assessing multiple dimensions and implementing tools and processes to maintain these standards.
Dimension | Description | Example |
---|---|---|
Accuracy | Ensures data correctly represents real-world facts. | A patient’s recorded blood type differs from their actual blood type, leading to potential medical errors. |
Completeness | Ensures all required data is present and usable. | A shipping database missing delivery addresses prevents order fulfillment. |
Consistency | Ensures data is uniform and does not conflict across systems. | An inventory system shows an item as "in stock," but the warehouse system lists it as "out of stock." |
Timeliness | Ensures data is updated and available when needed. | Delayed stock price data in financial trading can lead to significant losses. |
Validity | Ensures data adheres to required formats and rules. | An online form allows inconsistent birthdate formats, causing processing errors. |
Uniqueness | Ensures each data entry appears only once, avoiding duplication. | A CRM system contains multiple profiles for the same customer, leading to redundant communication. |
The six most used Data Quality dimensions with examples
1. Accuracy
Accuracy refers to the correctness of the data. It is considered accurate when it reflects real-world values or attributes it is supposed to represent. Inaccurate data can lead to incorrect conclusions and decisions.
- Example: In a sales dataset, accuracy means that every value in the “amount” column equals the actual amount charged to the customer.
- Improvement: Verify data against authentic references or actual entities to ensure it maintains accuracy throughout its lifecycle.
2. Completeness
Completeness assesses the presence of all required data elements within the dataset, without missing or omitted values. Data should be comprehensive and contain all necessary information for its intended use.
- Example: A salesperson requires customer contact information to follow up. Missing phone numbers make the data incomplete.
- Improvement: Set standards for the minimum information required and conduct regular checks to ensure completeness.
3. Consistency
Consistency is about the absence of conflicts or differentiations between different data elements, either between different datasets or within a dataset. Data should be consistent both within itself and with other related data.
- Example: If an employee’s termination date differs between HR and payroll systems, the data is inconsistent.
- Improvement: Regular audits and reconciliation processes can help maintain consistency across datasets.
4. Timeliness
Timeliness refers to the relevance and currency of data, ensuring it is up-to-date and suitable for decision-making. Timely data is updated and important for specific use cases. Outdated data can be misleading and result in poor decision-making.
- Example: Real-time fraud detection systems need timely data updates to function effectively.
- Improvement: Implement real-time or scheduled data update mechanisms to ensure the data is timely.
5. Validity
Validity ensures that data adheres to rules or standards, confirming its appropriateness for the intended purpose. Valid data conforms to predefined formats, values, and ranges essential for ensuring its utility and reliability.
- Example: Postcodes must adhere to a specific numeric format to be considered valid.
- Improvement: Regular validation checks and adherence to industry standards are essential for maintaining validity.
6. Uniqueness
Uniqueness assesses whether a dataset contains duplicate records or not. It involves identifying and eliminating repeated or redundant information, making sure that each data entry is distinct.
- Example: Duplicate customer records can lead to incorrect conclusions in analysis.
- Improvement: Identifying overlaps and using data cleansing and deduplication techniques to maintain uniqueness.
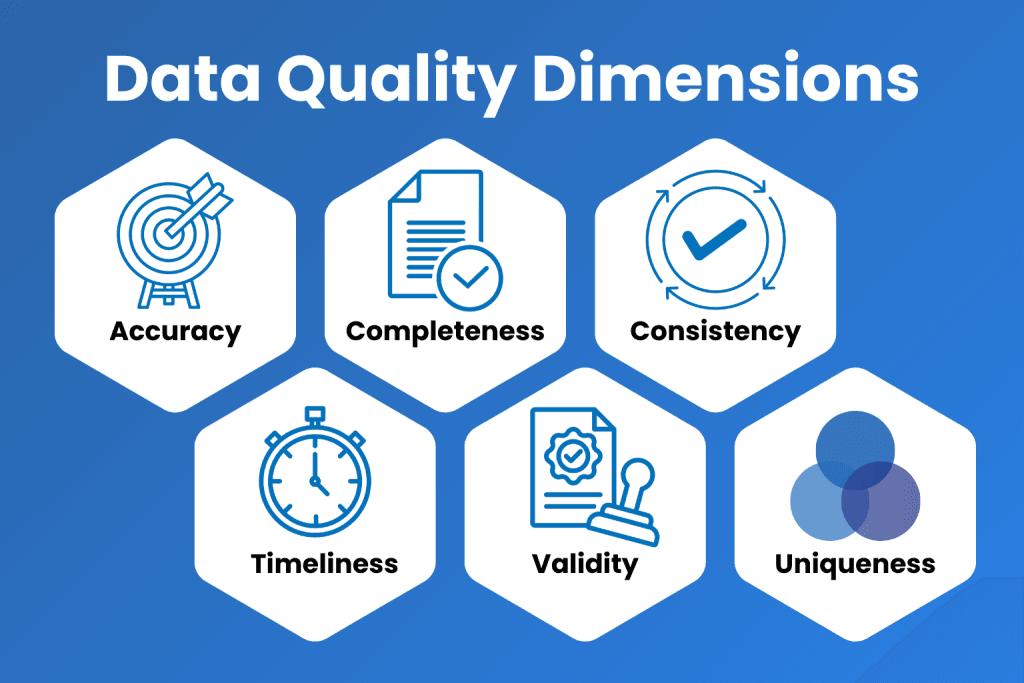
Additional DAMA-DMBOK Recognised Dimensions
In addition to the most commonly used dimensions, DAMA-DMBOK describes several others that offer valuable insights. These complementary dimensions help you assess Data Quality from different angles—supporting a more complete and nuanced view of how data performs across your organisation.
7. Integrity
Focuses on structural integrity within data relationships.
Example: Sales orders refer to customers that exist in the customer table.
8. Precision
Measures the level of detail in numeric or textual values.
Example: Latitude and longitude fields are stored with consistent decimal accuracy.
9. Availability
Indicates whether data is accessible when and where needed.
Example: A finance team can access daily revenue data before 9 a.m.
10. Portability
Reflects how easily data can move between systems without losing meaning.
Example: A dataset exported from SAP to Power BI retains all field mappings.
11. Auditability
Describes whether data changes are logged and traceable.
Example: Every update to a record includes a timestamp and user ID.
Implementing Data Quality checks
If your organisation has defined the most important Data Quality dimensions and is looking to start implementing Data Quality checks, there are roughly 5 steps to take. Of course, the whole process to implement Data Quality checks is a bit more complicated, but the steps below will give you a good head start:
- Define Data Quality standards: Establish clear criteria for each Data Quality dimension relevant to your organisation.
- Automated Data Quality tools: Utilise automated tools for data validation, cleansing, and monitoring.
- Regular audits: Conduct regular audits and quality checks to identify and rectify issues.
- Training and awareness: Train staff on the importance of Data Quality and how to maintain it.
- Feedback mechanisms: Implement feedback loops to continually improve Data Quality processes.
Curious on how to start implementing Data Quality checks within your organisation? Check our Data Quality services or get in touch with us!