Data Quality
Organisations should view data as an asset. Data is used as an input for many organisational processes, from basic customer service to complex machine-learning based applications. No matter how you use data, it should be of good quality and fit for purpose. Thoroughly analysing the quality of data is important because some issues can easily go unnoticed.
Following the ‘garbage in = garbage out’ principle, high quality data is essential to ensure success as a data-driven organisation.
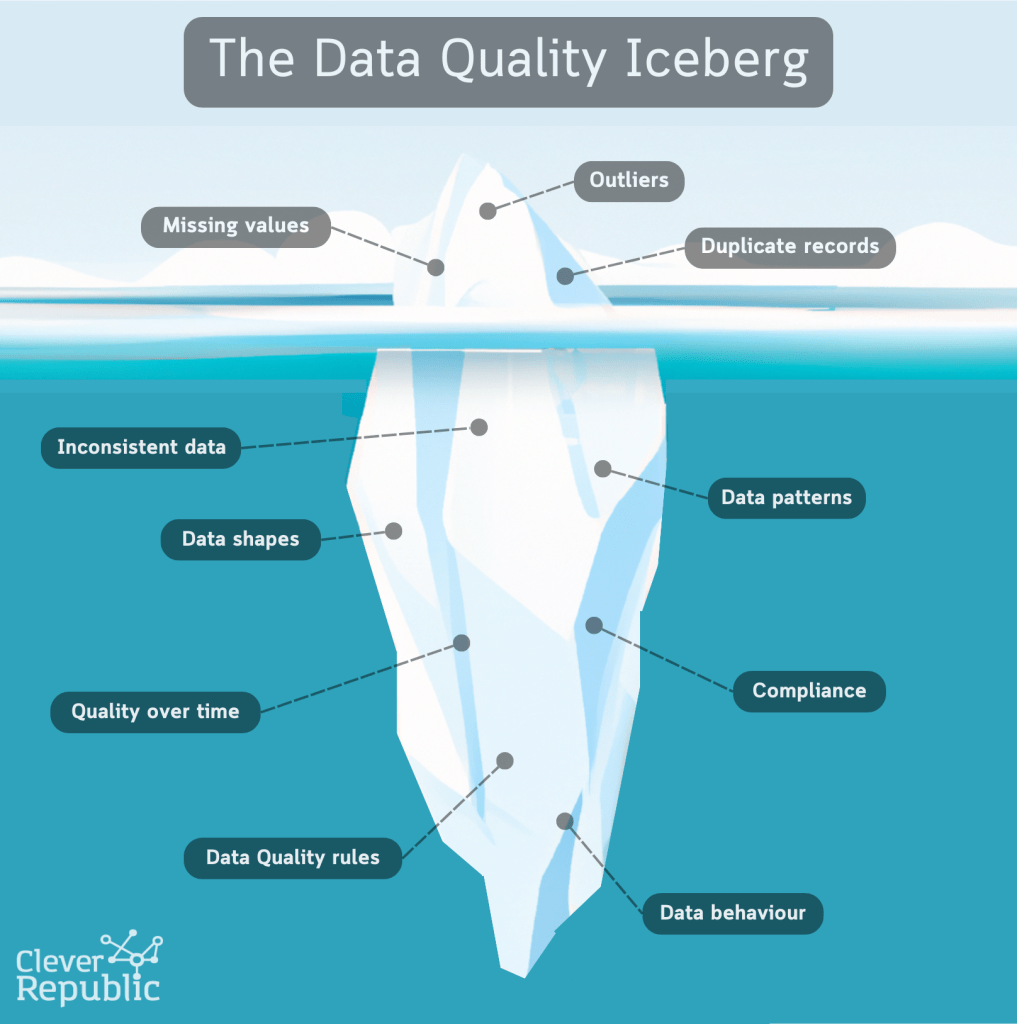
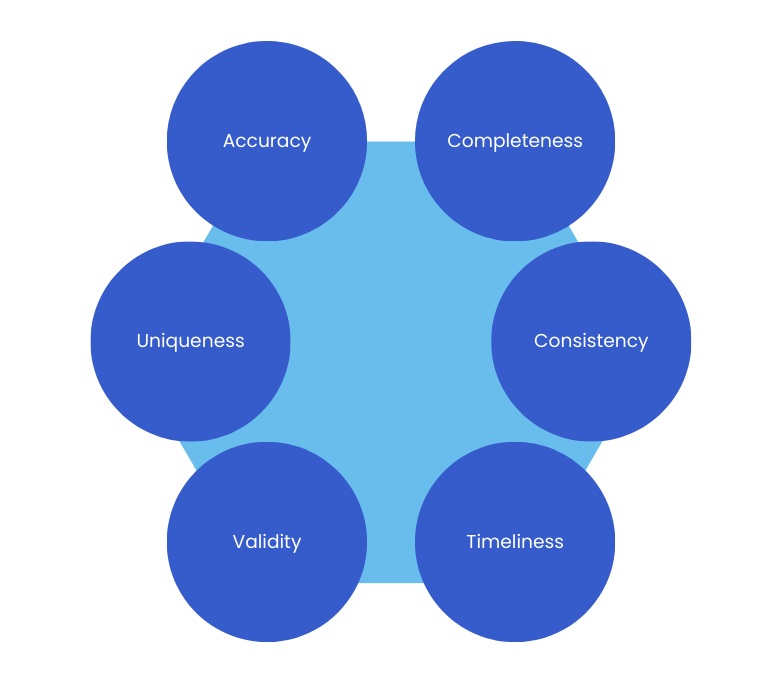
What is Data Quality?
To put it simply, Data Quality involves the continuous process of identifying critical data, defining what rules data should follow to be fit for purpose, identifying data issues based on these rules, and finding & eliminating the root cause of low-quality data.
The quality of data is often measured on multiple different Data Quality Dimensions. The most common ones are accuracy, completeness, consistency, timeliness, validity, and uniqueness.
Our services
Tools
Select Data Quality tooling that fits your needs, and make sure it is implemented in the right way.
Strategy
Lay a strong foundation for your Data Quality initiative with a Data Quality framework or internal Data Quality policy.
Training
Educate stakeholders on tool usage, writing of Data Quality rules, and best practices.
Blogs on Data Quality
Key Data Quality Management Capabilities
Data Quality Tools
Data Quality: A Journey Through Time
Common Questions
Data quality refers to the accuracy, completeness, consistency, timeliness, validity, and uniqueness of data within a dataset. High data quality ensures that information is reliable and suitable for its intended use, supporting effective decision-making and analysis.
Always start with an assessment of your Data Quality requirements and scope. What processes are critical? And what data is consumed in critical processes. It is helpful to establish a Data Quality policy or framework that defines what data in your organisation is critical, which Data Quality dimensions are used, and how rules are executed.
For middle- and large sized organisations, a dedicated Data Quality tool is essential to improve Data Quality at a large scale. Data Quality tools help you with data profiling, implementing Data Quality rules, and provide comprehensive dashboards.
Having good quality data entails compliance to regulations, but also making sure it is fit for purpose. It increases trust in analysis and reporting in your organisation. Better quality data ultimately leads to better business decisions.
The six most used Data Quality dimensions are:
- Accuracy: the correctness of data.
- Completeness: the presence of required data elements (no missing values).
- Consistency: the absence of differentiations between data elements.
- Timeliness: the relevance of data and presence of up-to-date data.
- Validity: data adheres to rules and standards, and is fit for purpose.
- Uniqueness: the absence of duplicate records.
Interested in our Data Quality services?
Could you use some help with the implementation of Data Quality within your organisation? Are you looking for help in setting up your strategy? Or how to implement a Data Quality tool?
Contact us for more information or to request a demo.